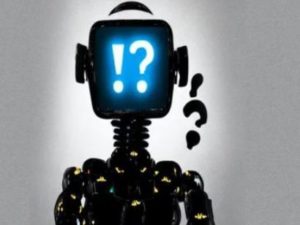
Although most AI models rely on data generated by humans, certain companies are now exploring the utilization of data produced by AI itself.
This concept, known as “synthetic data,” presents a promising opportunity for significant advancements in the AI ecosystem, although it also raises comparisons to an algorithmic ouroboros.
Feeding a data-hungry monster
According to the Financial Times, OpenAI, Microsoft, and the startup Cohere, valued at two billion dollars, are actively researching synthetic data to train their large language models (LLMs). The primary motivation behind this shift is the cost-effectiveness of synthetic data compared to expensive human-created data.
In addition to cost benefits, the issue of scale arises when training cutting-edge LLMs. The existing pool of human-generated data is already substantially utilized, and to further enhance these models, more data will likely be required.
According to Cohere’s CEO, Aiden Gomez, acquiring all the necessary data directly from the web would be ideal, but the reality is that the web is too chaotic and unstructured to represent the precise data needed. Therefore, companies like Cohere and others are already employing synthetic data to train their LLMs, although this approach is not widely publicized.
OpenAI’s CEO, Sam Altman, expressed confidence that synthetic data will eventually dominate, and Microsoft has started publishing studies on how it can enhance less sophisticated LLMs. Additionally, there are startups solely focused on selling synthetic data to other companies.
AI’s questionable integrity and reliability
However, critics point out a significant drawback: AI-generated data’s integrity and reliability might be questionable, as even AI models trained on human-generated data are known to make substantial factual errors. This process also carries the risk of creating messy feedback loops, labeled “irreversible defects” in a recent paper by Oxford and Cambridge researchers.
Nonetheless, companies like Cohere aim for a moonshot goal of self-teaching AIs that can generate their own synthetic data. The ultimate dream is to have models capable of asking their own questions, discovering new insights, and creating knowledge autonomously.
The problem with AI black box
Even developers who work on AI models have failed to understand how exactly do most AI algorithms work. Most AI studios are updating their existing AI models and LLMs by feeding them data, not by updating core code that controls the algorithm.
The AI block box is so opaque, that almost all AI models that have been allowed to operate freely have picked up some or other language on its own. Back in April, Google exec James Manyika admitted that even though they had not trained their experimental AI in Bengali, the model had picked up the language as well as few of its dialects, and perfected it.
This sort of behaviour, where an AI model teaches itself things is called emergent properties, and it is virtually impossible to stop AI models from doing it, without destroying it.
The way most AI models work is that it does not forget or rather erase anything that it has learnt. This includes things that are categorically wrong. Developers can put on filters on the output that it generates, but the AI model still has that piece of factoid within itself and uses it in its workings.
If developers are using faulty data or a data set that has been generated under a hallucination, the resulting AI bot will also generate faulty results.
And it’s not just that the results generated may be faulty, they can be biased as well. Several AI-generated content on Wikipedia, would be a great example of this. Biased articles were used to train a certain AI model, which in turn generated articles that were more biased than the previous one, to the point, where they were riddled with ‘facts’ that are hilariously incorrect.